[Research] Heart rate variability (HRV), training load and subjective data
Using HRV4Training for data collection
In Autonomic Response to Training Load through Daily Control of Heart Rate Variability in Female Athletes, Juan Jose Palos and co-authors look at the relationship between heart rate variability (HRV), training load, and subjective data in a professional football team over 6 weeks, using the HRV4Training app (you can find the poster here).
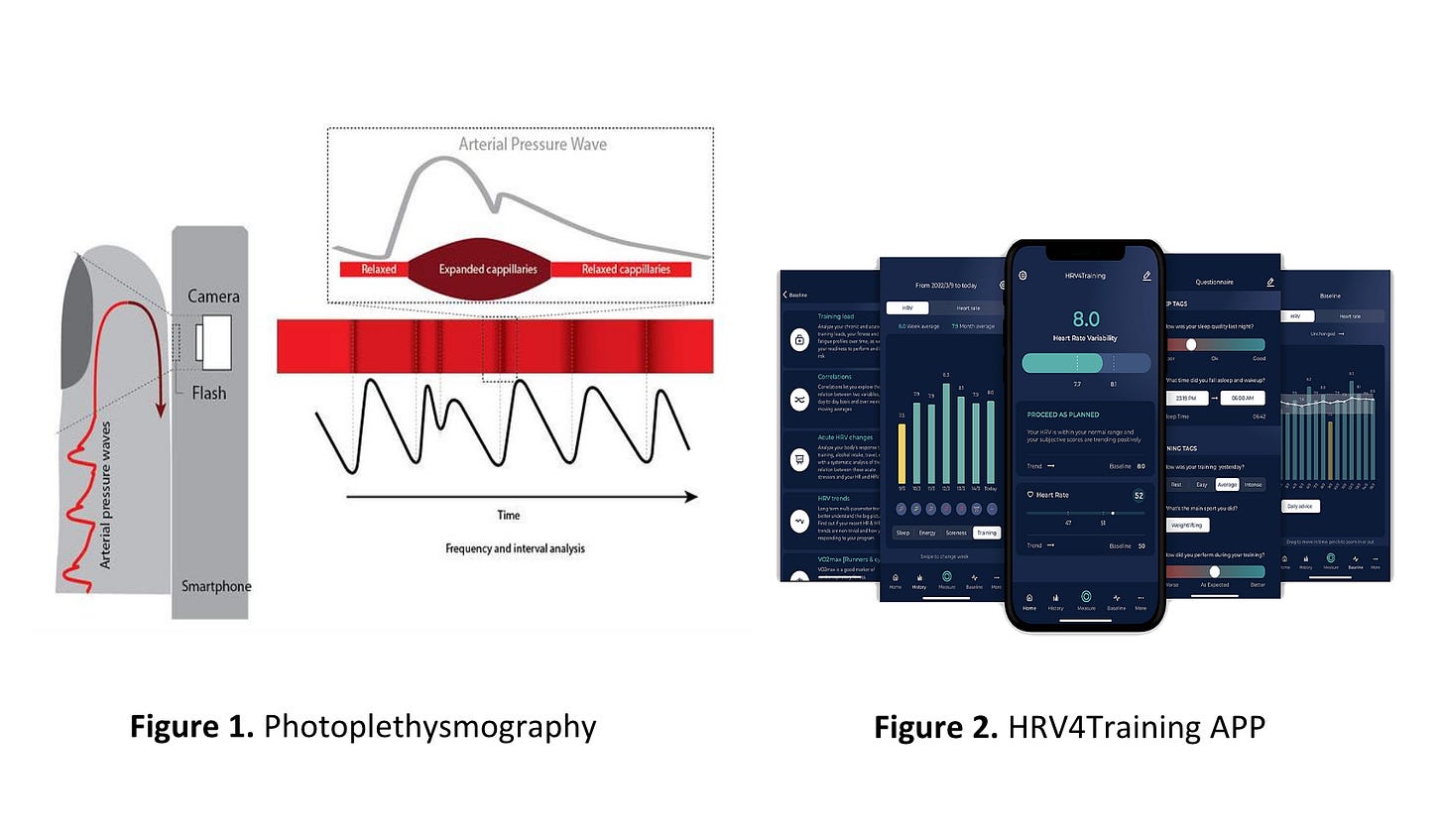
This is an interesting analysis fo HRV data, which I will try to break down below in relation to various aspects I’ve previously discussed in my blog, hoping you can find some useful pointers.
In particular, the authors report no correlation between HRV and training load, something that I would normally expect, as I discuss in my blog “Should Heart Rate Variability (HRV) be associated with training load?“, which you can find here.
In short, it is a common misconception that HRV should track training load, for example reducing when training load is higher. HRV is all about individual responses. An ideal response to high training load (intensity and or volume) is a stable HRV, with a quicker rebound for more fit individuals, as I discussed in this other blog post. Only in case of issues (e.g. a poor response to increased load), a reduction in HRV will be present, and therefore a stronger (negative) correlation between HRV and load will also be present (this could be the case when going from the off-season to the pre-season, context always matters).
How about subjective data?
Here is where things get more interesting. For example, HRV was statistically associated with self-reported indicators of fatigue, muscle soreness, training motivation, mental energy, and sleep quality (all metrics you can log subjectively in the HRV4Training app, via the questionnaire that you fill in after your morning measurement). The associations are all in the direction you’d expect (higher HRV = higher motivation, higher mental energy, higher sleep quality, while lower HRV = higher fatigue, muscle soreness). These associations make sense as both the morning physiology and your subjective feel are outputs, i.e. assessments of the impact / result of training, hours after the fact, to establish your response, which should be tightly coupled to your readiness or recovery. Ironically, readiness or recovery scores in werables are in fact relying on inputs, i.e. your behavior, such as activity levels and sleep time (as well as physiological data often collected too close to the stressor), which confounds the score and makes the data less representative of your response (you can learn more here and here).
As many coaches remind me, the questionnaire might just be the best part of the HRV4Training app, as it prompts a moment of reflection, increasing self-awareness, before looking at the physiological data (the exact opposite of what you get when using wearables, sadly).
I will add here that despite these associations, even the relationship between HRV and subjective data is not to be taken for granted, depending on the training stimulus and other contextual data.
For example, after a hard session, I might be sore, but if my HRV is back to normal the following day, this quick rebound - which could be due to a high level of fitness, etc. - is somewhat decoupled from my subjective feeling of soreness and fatigue.
This is exactly why these three parameters: load (as context, i.e. the work done), subjective data (how we feel) and objective physiological data representing internal load (e.g. HRV, or our response, when measured at the right time, i.e. in the morning), are all key to try to capture the complex interplay between training stimulus and response, so that we can better individualize training and improve performance in the long run.
If my training load is high and my subjective feel is not so good (but temporary), but my physiology is normal, I am heading in the right direction.
If you use HRV4Training Pro, you can track resting physiology, training load, and subjective data, with respect to your own normal ranges, in the Overview page.
You can also see automatically computed correlations for various combinations of parameters, which might help you understand how the different metrics are trending over time.
HRV4Training Pro: Overview
HRV4Training Pro features an overview page that aims at easing the interpretation of HRV data for your own analysis as well as your team data. The page shows the normal range, daily and weekly averages for HRV and heart rate data. You can optionally highlight each recording with different annotations, and add training load and subjective parameters as c…
Marco holds a PhD cum laude in applied machine learning, a M.Sc. cum laude in computer science engineering, and a M.Sc. cum laude in human movement sciences and high-performance coaching.
He has published more than 50 papers and patents at the intersection between physiology, health, technology, and human performance.
He is co-founder of HRV4Training, advisor at Oura, guest lecturer at VU Amsterdam, and editor for IEEE Pervasive Computing Magazine. He loves running.
Social: