Talk for the Royal Society of Medicine
Making use of wearables for sleep and physiology tracking, November 2023
Yesterday I gave a talk at the Royal Society of Medicine about wearables for physiology and sleep tracking.
This was a new talk for me (which, in slightly different forms, I will also do in other events this week and in the next months), as I normally mostly cover heart rate variability (HRV) alone.
I've been thinking about these topics the whole summer: trying to find ways to communicate how wearables can be useful and also their limitations eventually, I decided to focus on the following:
Measured (few things, reliably) vs estimated (lots of things, not so reliably), with examples
Estimates of actual things (sleep time, stages, etc. - potentially meaningful) vs estimates of made-up things (readiness, recovery, sleep quality, etc. - meaningless), also with some examples
Specific guidelines for sleep tracking wearables (validations, bias, practicalities, more on this in an upcoming paper with a group of experts)
The general idea that maybe we should focus less on e.g. estimates of sleep stages and more on what wearables enable us to do with a higher degree of accuracy: looking at changes in autonomic activity, in free-living, longitudinally (something not part of what we could do with previous generation clinical-grade sleep trackers like e.g. the now discontinued actiwatch)
In this blog, I elaborate a bit more on each point, covering part of what I have discussed in the talk, as well as some of the questions that I was asked, which I think are quite relevant to further clarify a few aspects.
Goal of the talk
Wearables are out there, and patients and researchers are using them. At the same time, clinical tools are being discontinued (e.g. the actiwatch), and wearables offer a great platform for tracking data continuously, unobtrusively, at a large scale. However, they are also black boxes with validity concerns.
I am getting some pushback from wearables manufacturers on this talk, but I think it is short-sighted. The better we communicate strengths and limitations, the more people can benefit (and the companies themselves will benefit in the long run). Otherwise, we just have fanboys ("wearables are great") and detractors ("wearables are not good for anything"), which is far from the truth, and as such, far from helping people make effective use of wearables.
I've seen many going from fanboys to detractors because they were disillusioned after "something" was clearly not correct (e.g. an estimate of calories for a day, or an activity, or "stress", something trivial typically). This would not happen if the limitations were more clear and the focus was more on what can actually be measured with high accuracy (e.g. even just resting heart rate and how it changes in relation to stressors).
Work in progress, read on in the meantime.
A framework for wearables data
The main point I am making for part of the talk is the distinction between what is actually measured by a wearable, and what is estimated. I cover this in greater detail in the blog below:
Briefly, when we measure something, we determine its exact value give or take a measurement error. We have a sensor designed to measure that thing that we are interested in. An example is heart rate via ECG or pulse rate via optical measurements.
When we estimate something, we take a guess. We can take simple guesses (maximal heart rate based on age), or more complex guesses (sleep stages based on a machine learning model combining accelerometer, heart rate variability and temperature data), but we are still guessing.
I draw a line between things that we can measure thanks to a sensor that was designed for that purpose and things that we are trying to guess because they are somewhat related to the other parameters that the sensors can measure.
Measurements
Measurements are of course preferable, but are not perfect either. In the talk I cover accuracy and how to determine it (eg comparing against a reference device), and the importance of when we measure, which is multifactorial and deserves a few more words.
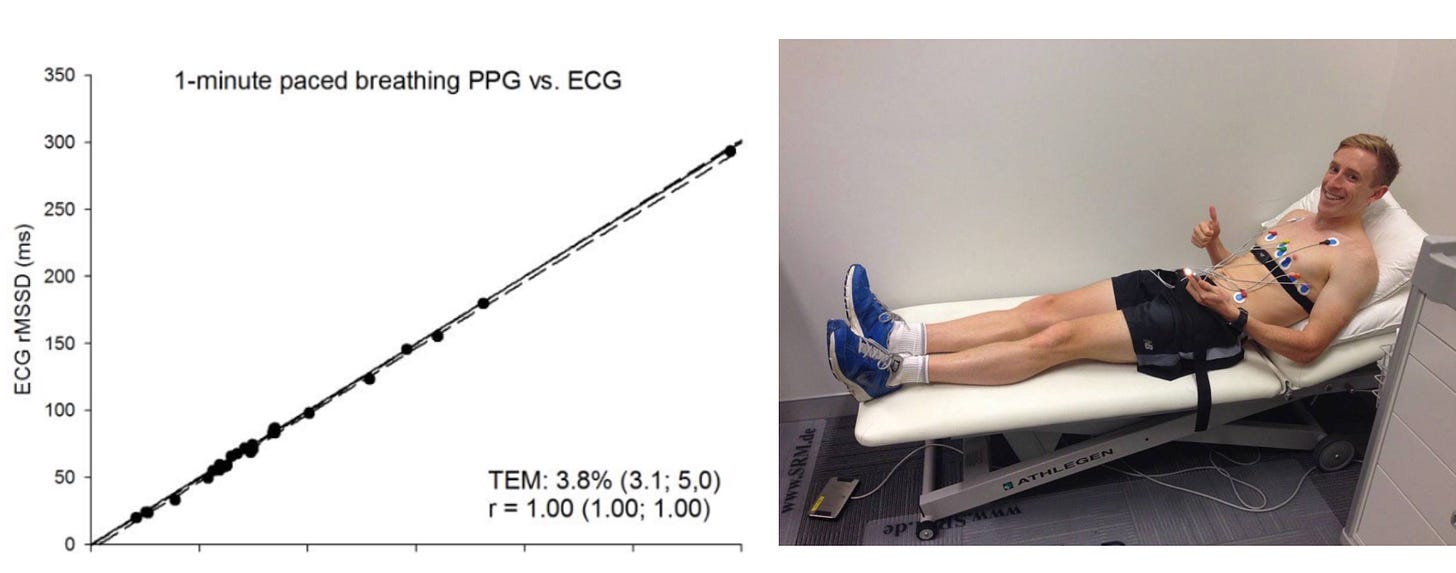
In particular, when we measure, impacts accuracy and interpretation of the data.
When looking at accuracy, we can see how results are a bit all over the place, depending on the context. We cannot even say that a device is better than another, as they outperform each other in random activities. When do the sensors work well consistently? Only when there is no movement, at complete rest, during sleep. This is important to remember: data is good and consistent between devices only if you are not moving at all (the error is more than doubled by just "sitting").
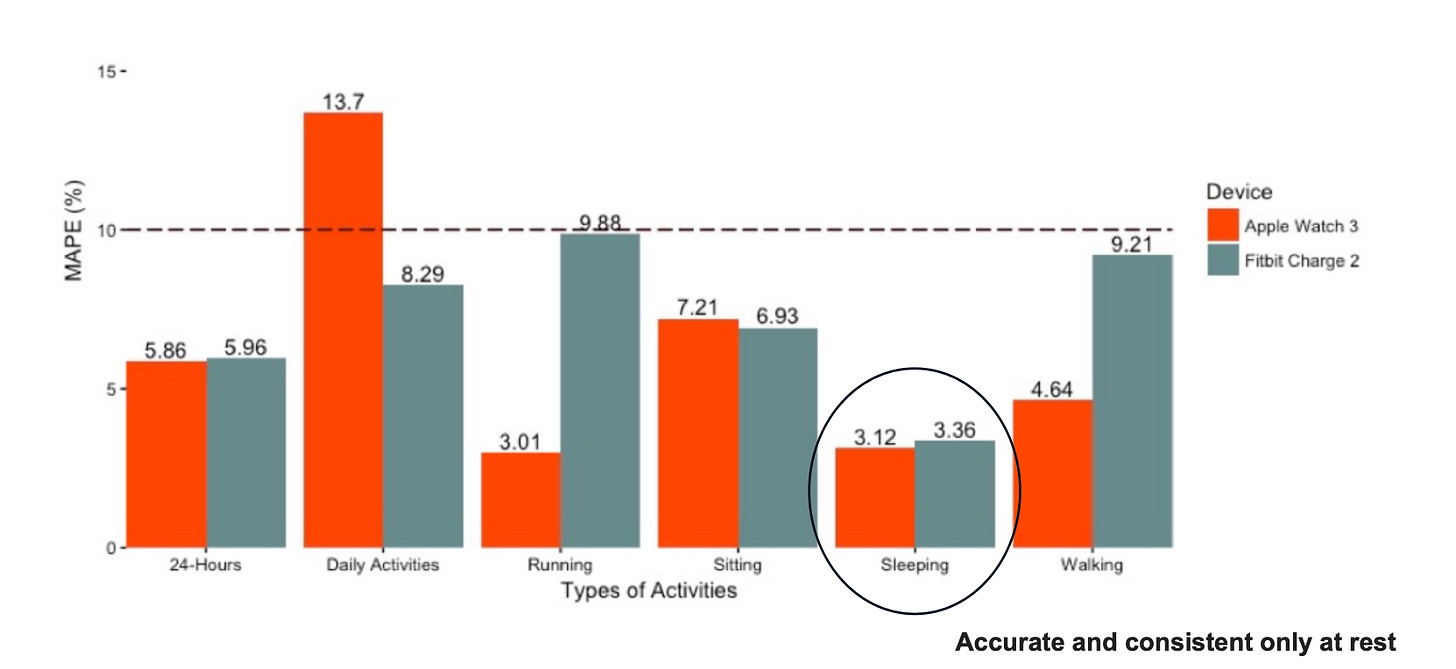
In terms of interpretation, we need to keep in mind that physiological meaning is impacted depending on when we measure, for example, sampling sporadically even if accurately provides no value in terms of assessing physiological stress using heart rate or HRV (details in this blog). Similarly, measuring HRV during the day after you had some water also makes no sense even if the measurement is accurate, because HRV increases with no link to stress (see also this blog).
Once we have an accurate measurement, and we take it at a meaningful time, then we look at how to interpret it, which normally is about adding a frame of reference (ie a normal range), and looking at deviations from our normal. This is necessary as many variables do not really have a population-based frame of reference that would work for each one of us. To learn more, see below.
To wrap up the measurements section: make sure to pick a wearable with a low error for the parameter you are interested in. Measure that parameter at the right time, which impacts both data quality and its physiological meaning, and assess deviations from an individual's normal range, to interpret the data.
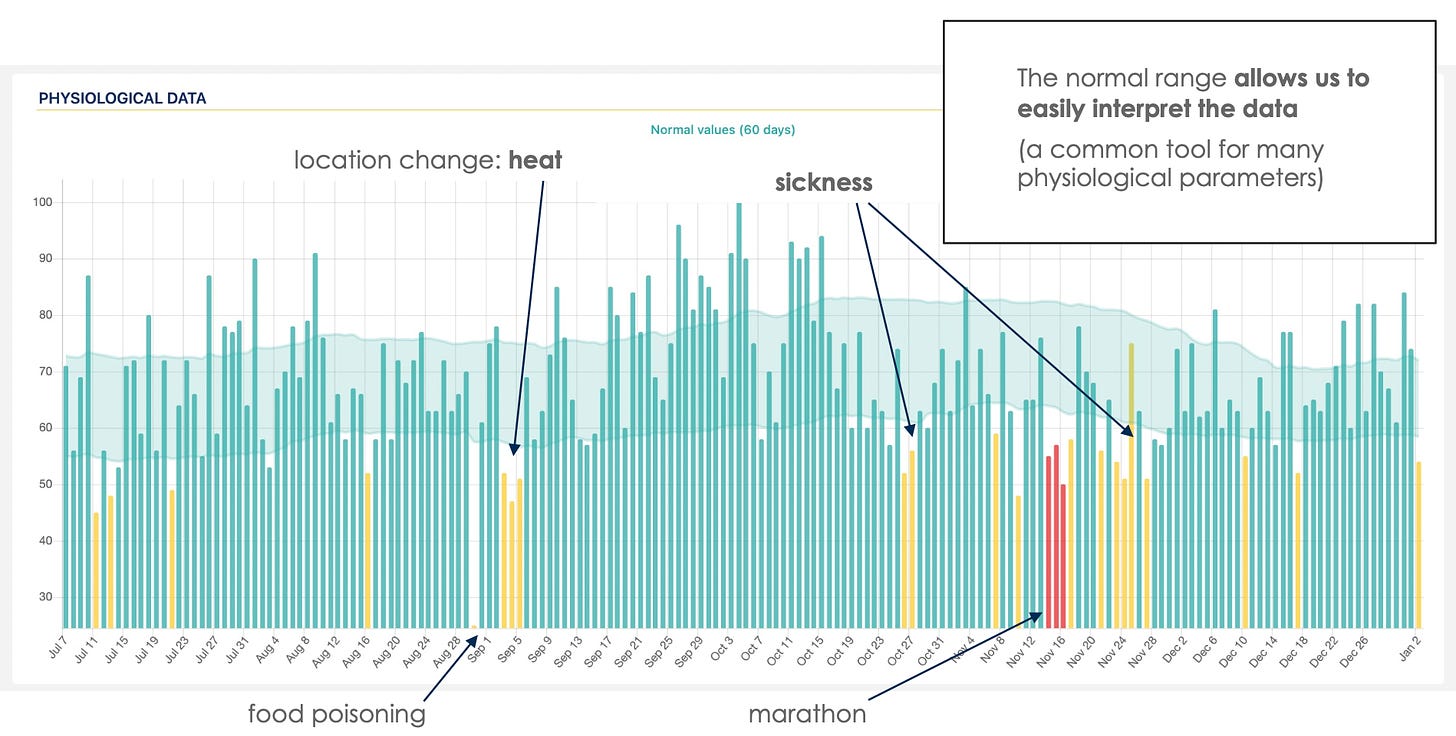
Estimates
When I talk about estimates I like to discuss a few points:
Do not generalize from measurements: we should never assume that because a device is good at measuring something (e.g. an Apple Watch can measure resting heart rate with high accuracy) it is also good at estimating something (e.g. an Apple Watch is not good at estimating SPO2). See the figure below.
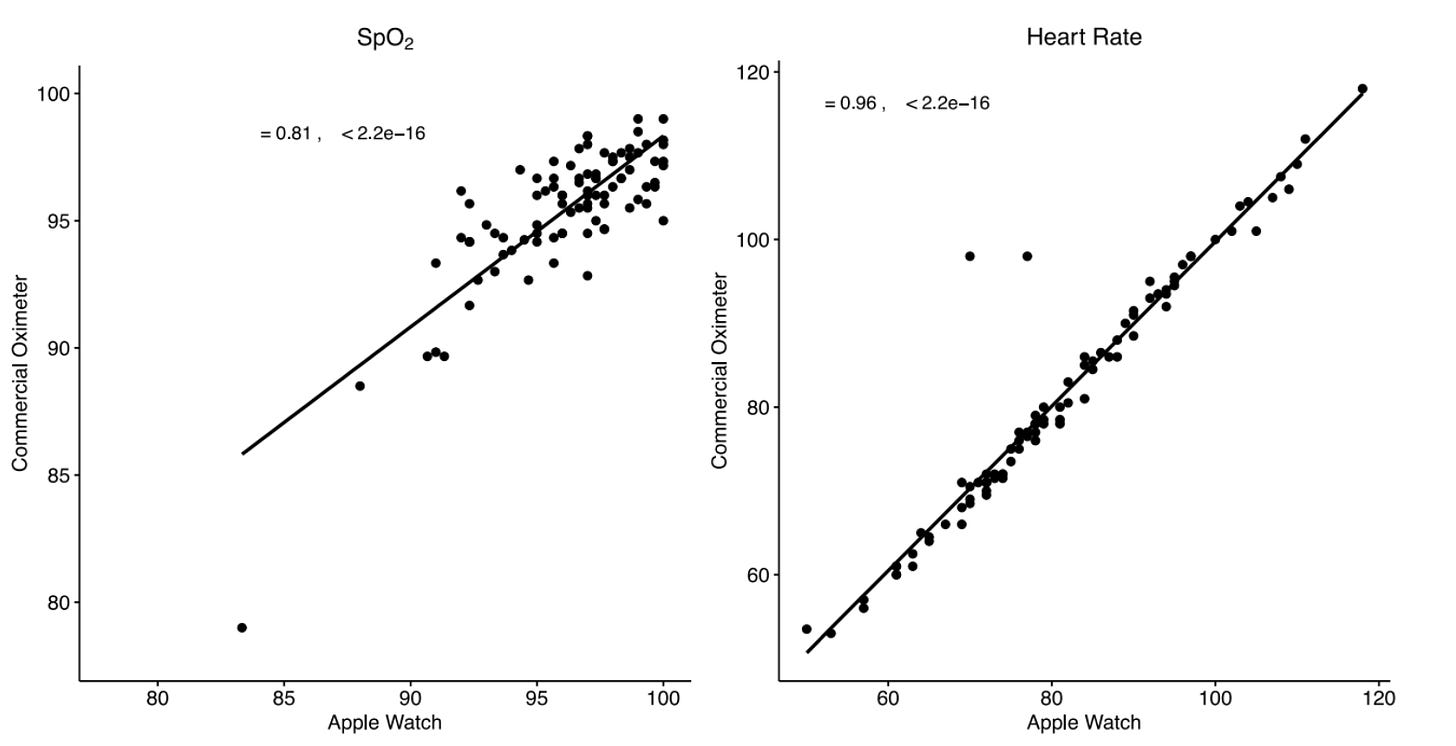
Error compounds: estimates are guesses and when we make an estimate on top of an estimate (as often wearables do), error compounds. If we measure heart rate, which also might have some error (artifacts), then we use it to estimate sleep stages, which typically have quite a large error, then we use these sleep stages and activity (with an error too, for example, if our activity did not involve much wrist motion) to estimate recovery or readiness, then we have put errors over errors over errors. What do we expect?
Validations or inconsistencies in output. As wearables move faster than academia, we might also have no published literature yet. A good heuristic in my view, is to compare or look for comparisons of multiple devices. If the output of multiple wearables for a given parameter is inconsistent, it is a clear red flag that you should not be bothering with this parameter: we are unable to estimate it reliably.
To clarify these points, I use the excellent work of Peter Tierney as an example. When looking at measurements, heart rate between devices is the same:
When moving to estimates, let's call them simple estimates, like sleep time, we can have large errors on any given day (e.g. more than an hour), but the trend seems to be captured reasonably well (for this person - keep in mind that individual differences in behavior can cause issues, e.g. I can’t get any wearable to work properly because I read a kindle in bed):
When moving to more complex estimates, things seem to be all over the place, which makes me quite skeptical about our ability to get any meaningful insights with these devices (despite having developed and validated one of these models myself):
Known vs unknown parameters
I don't really like to talk about made-up things like readiness, recovery, sleep scores, body batteries, and all of that nonsense, but I do cover them since they are front and center in the apps.
The main point I make here is the one I covered in the blog below, and how these scores make assumptions instead of assessing your response, and as such, they are of very little value. Learn more, below.
Take a look at this tweet too, in case you are still in doubt.
Sleep tracking with wearables
This section of the talk is something I have added specifically for the audience of this specific conference since it was about sleep, but I would normally leave it out if I am discussing wearables more broadly.
In the context of sleep, I covered how to evaluate them (Bland Altman plots, epoch-by-epoch analysis, and how these methods still do not look at what could be the most important aspect: how the data tracks over time within individuals).
This content comes from a paper I co-authored with a group of sleep and technology experts, which will be out soon (more about this, later). In particular, I covered how regardless of the accuracy and the validations, we need to keep in mind sources of bias, and how the algorithm was developed: was the population of interest the same we are working with as clinicians or researchers? what happens when we look at minorities or chronic health conditions or medications that impact ANS activity.
This is similar to the VO2max example discussed below (and in this tweet). If the predictor changes (e.g. HRV because of medication), then the estimated sleep stages will also change, but these changes might not have anything to do with actual changes in sleep stages (which might differ, but not in the way that the wearable would reflect). The relationship between input and output in these models can easily break for a number of reasons.
I have also covered practical considerations and how to select a device based on our necessities: granularity of the data, raw data availability, evaluations in similar populations, financial constraints (subscriptions), need for internet connection, comfort, battery life, privacy, updates, and most importantly: how the data influences the user since they cannot typically be blinded to it for too long or the data might be lost if not synchronized.
Where to go from here
When it comes to wearables, very few metrics are measured, and many are estimated (either known parameters or unknown or made-up scores). Given issues with measurements outside of complete rest, and how errors compound, my recommendation is to spend your time and energies on the few parameters that can be actually measured.
Minimize error (e.g. looking at resting data) and maximize physiological meaning (e.g. looking at well-contextualized data, e.g. night or first thing in the morning). The more we move away from resting measurements, the more noise / error we introduce.
Wearables are great (in case it wasn't clear yet).
They allow us to easily collect longitudinal data, with great potential for the individual in many applications related to sleep, health, and performance.
However, my recommendation is to always ask yourself: is the parameter I am interested in actually measured by this device, or is it estimated? if it is estimated, what is the degree of error? Can I find a validation? If not, when using multiple wearables to look at this specific parameter, are results consistent? If not, maybe take the output with a grain of salt. Be very cautious implementing interventions or assessing their effectiveness and changing behavior based on the data you have captured with wearables. It is extremely unlikely that if you change something and see a reduction in eg REM sleep, you actually had a reduction in REM sleep. I hope this is clear by now.
Finally, in the context of sleep tracking, as we move away from clinical-grade sleep trackers that are being discontinued (well, glorified accelerometers like the actiwatch), we do have an opportunity to start going beyond actigraphy.
My recommendation is to focus on measurements of autonomic activity and how these parameters change in health and disease, in relation to various stressors, within individuals, in free living. This is probably the best we can do currently with the most common wearables (fitbits, apple watches, oura rings, whoop bands, etc.).
Questions
the questions weren't exactly the ones I wrote below (if you were there, feel free to comment), but more or less it was on these lines. The answers are further elaborations of what I said there briefly.
Wearables are not medical devices, but indeed people are using them, and are coming to us (clinicians) with the data. Is there a trend into making wearables medical devices?
I think that there is a trend in validating more and more some of the features, some components might be also FDA approved. While the entire device remains a consumer device, there is a positive trend I think in the context of validating certain features. Thinking about this a bit more, I think that this is an even more important reason to be more transparent about what is measured, what is estimated, how not everything that comes out of a wearable should be trusted in the same way, etc.
If there is a bias, but the wearable can still estimate the variable accurately, is that okay? (example for VO2max)
This is not as simple as it seems. An important issue, which can be generalized as follows, and often arises when estimating parameters: if a change in behavior (eg training at different intensities, but you can think of other applications) modifies the relation between predictors (eg heart rate vs pace) and predicted (eg VO2max) variables, we might see spurious changes in the predicted variable. See also Alan’s tweet for an example.
You might think the estimate is not accurate in absolute terms but tracks well over time, in relative terms. This is what wearables manufacturers often say these days, as it is clear they are not getting the absolute value correct However, due to the issue above, not even relative changes are meaningful (this is not always the case of course, but beware that changes in behavior and therefore in predictors, might cause changes in predicted variables that have nothing to do with actual changes in that parameter, if you were to measure it). Whenever possible, measure, do not estimate, and do not rely or extrapolate too much based on wearables estimates. See also my example about sleep stages (predicted variables) estimation when taking medication that impacts heart rate or HRV (the predictors).
Sleep stages are validated when developing algorithms, hence they should allow us to look at relative changes over time, within individuals, even if we have inconsistent results at the group level (?).
This is maybe something I didn't explain well when going over Peter's slides. In particular, what we see in these slides (see above), is how within individuals, we fail to capture changes in sleep stages consistently. If one device tells you that your deep sleep is decreasing, and the other one says it is decreasing, when doing the same intervention, then what are we doing?
Do you also estimate 'recovery / readiness' in HRV4Training?
No, in HRV4Training we provide only physiological data as numbers. We do transform rMSSD to make it more user-friendly, but that's just HRV. The advice (messaging and color) does include your subjective questionnaire responses because of course, there is more to daily decisions than HRV. But no numbers are made up combining behavior and physiology, the way wearables do.
Note also that adding your subjective questionnaires in the decision-making is very different from adding your activity or sleep in the equation: subjective data collected in the morning, just like HRV, reflects the output of the system. Behavior (activity or sleep) reflects the input. The input should not deterministically impact the output (ie if you sleep less your readiness will always be lower, which makes no sense if your physiology or you subjectively have not been impacted).
What wearables do I use if any?
I have an Oura ring (as you know I work with Oura), which I wear only at night, as I have no use for a wearable during the day (for reasons I have discussed a few times).
In terms of assessing my response to stress and for decision making, I take my measurements in the morning, which I believe is the best time, while sitting (see why here) using HRV4Training and the phone camera, to measure resting physiology. However, I am also interested in seeing how night data captures physiological stress in somewhat different ways (which I discuss for example here).
Normally we are always told to keep everything up to date, but I recommended not to update devices when doing a study, isn't this inconsistent?
It is, but during a study, it is key not to update hardware, firmware and software, as otherwise the outcomes of interest might change simply becasue the algorithm is different, not because there is an actual change e.g. in our intervention. Unfortunately, this happens also all the time to users self-experimenting. Focusing on measurements (accurate ones), this would not happen.
There were some other questions, but here is what I could recall today.
Marco holds a PhD cum laude in applied machine learning, a M.Sc. cum laude in computer science engineering, and a M.Sc. cum laude in human movement sciences and high-performance coaching.
He has published more than 50 papers and patents at the intersection between physiology, health, technology, and human performance.
He is co-founder of HRV4Training, advisor at Oura, guest lecturer at VU Amsterdam, and editor for IEEE Pervasive Computing Magazine. He loves running.
Social: