Issues with continuous heart rate variability (HRV) measurements
The physiology, technology and data interpretation of a (falwed) emerging application
As it becomes easier and easier to capture HRV data (or at least PRV, the version of HRV captured when using optical methods via PPG), it follows that the interest grows in measuring HRV all the time.
In this blog, I’d like to argue against this approach or at least provide some pointers related to why we should be very cautious.
Some of my reasons have to do with physiology, others with the technology, and yet some more with the interpretation of the data.
Let’s get to it then.
Physiology
What’s HRV?
HRV is a measure of the variability between heartbeats. Under certain conditions, this variability is mostly due to the parasympathetic nervous system, which modulates heart rhythm in response to different factors, such as stress. Note that other factors impact HRV, for example, the baroreflex, mechanical stimuli, and hormones.
Under certain circumstances, HRV is a decent proxy of the stress response. These circumstances are: measurements taken under controlled conditions, at rest, while breathing normally, and far from stressors. This is why HRV is normally measured first thing in the morning (or possibly, during the night, if we use several hours of data not to be fooled by sleep stages and other aspects causing variations in HRV during the night). Outside of these circumstances, HRV might not reflect what we are interested in (i.e. parasympathetic activity), and as such, might be meaningless.
To begin with, the autonomic functions captured by HRV are the ones that regulate heart rhythm - by definition. However, the parasympathetic system has other functions that are not captured by HRV measurements. For example, digestion is a highly parasympathetic state, hence after eating you’d expect to see high HRV if HRV was representative of parasympathetic activity. However, since heart rate is increased for the extra work required during digestion, HRV will be suppressed. A suppression in HRV (or even - if we wanted to extrapolate - in parasympathetic modulation of heart rhythm), does not mean that overall the body is not in a highly parasympathetic state, in this case. It follows that continuous measurements, or any measurement outside of the morning routine, would fail to capture this state correctly. Only by measuring far from meals, you’d capture a state that is not confounded by changes in parasympathetic activity that cannot be captured using HRV.
HRV can be considered a marker of parasympathetic activity only under certain circumstances. Why do we care about parasympathetic activity? Because it is part of the stress response, hence when we measure HRV (under certain circumstances), we can use it as a marker of the stress response (e.g. a lower HRV might indicate a poor response to stress).
In fact, variability in beat-to-beat data (HRV), is not only due to parasympathetic influence on heart rhythm, but it is typically due to a combination of the following: breathing and the baroreflex (how heart rate and blood pressure adjust), parasympathetic activity, hormonal changes, mechanical stimuli (stretch receptors), and possibly more.
So what are the certain circumstances I was talking about? When we collect data according to best practices, intentionally, using protocols such as measuring far from stressors, while rested, while breathing normally, without swallowing, yawning, talking, etc., then only then, can we hope that what we capture actually has something to do with parasympathetic activity. When we measure HRV outside of these protocols, i.e. when we measure continuously, or when we do not account for the many confounders that impact HRV, we are not looking at parasympathetic activity anymore.
We are just looking at HRV. You can always measure something (e.g. you can always measure HRV), but its meaning is not the same: HRV results from many processes, and if parasympathetic activity is not the main one due to confounding factors or else, then what we are looking at has little (or nothing!) to do with stress, which is the only reason why we looked at HRV in the first place.
If HRV is changing but this change is not due to the stress response, but only to e.g. a change in blood volume because we drank plenty of water, or has doubled because we swallowed saliva, who cares?
Examples of irrelevant confounders
Outside of these well-defined morning protocols (or the night), any sort of irrelevant factor can impact HRV in ways that are not meaningful or actionable. Below I report a few very common ones, that happen with high frequency (e.g. in the order of minutes), making the whole application of continuous stress monitoring highly ineffective.
Drinking water
The figure below is from research carried out by James Heathers. You can see a clear dose-response relationship between HRV and drinking water. As most people seem really quick to associate behaviors or other factors to changes in HRV as if they were positively or negatively impacting our health, remember that this is often not the case, as shown in the dose-response between HRV and drinking some water.
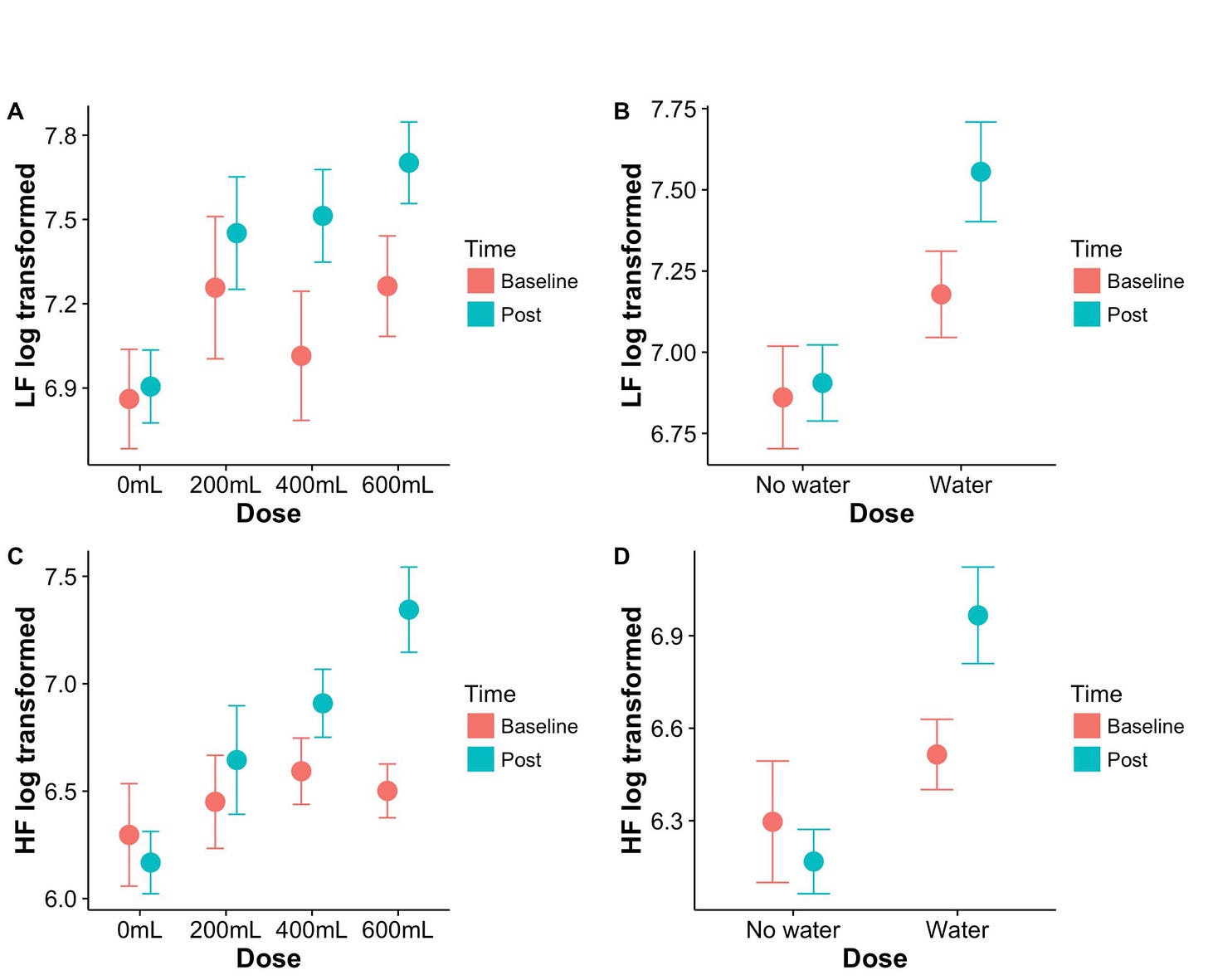
No, you are not less stressed because you had more water. But if you do drink water, then expect HRV data to be impacted for an hour, a long-lasting artifact.
Swallowing saliva
Another one that sort of kills it for this real-time application is swallowing saliva.
Swallowing temporarily increases heart rate, hence it is something not to do when you take your measurement, as it would cause a very large difference in HRV. This is obvious to anyone who has actually looked at some data: the RR intervals time-series changes, there's an abrupt suppression for a few seconds, the regular rhythm that is normally mostly mediated by your breathing is disrupted, and as a result, HRV suddenly becomes quite high and more variable over time (unfortunately, you can never see the actual data in a wearable, just ‘estimates’).
Below is some data where you can see the impact of swallowing saliva on RR intervals (beat-to-beat data used to compute HRV), and on HRV (the rMSSD feature) itself:
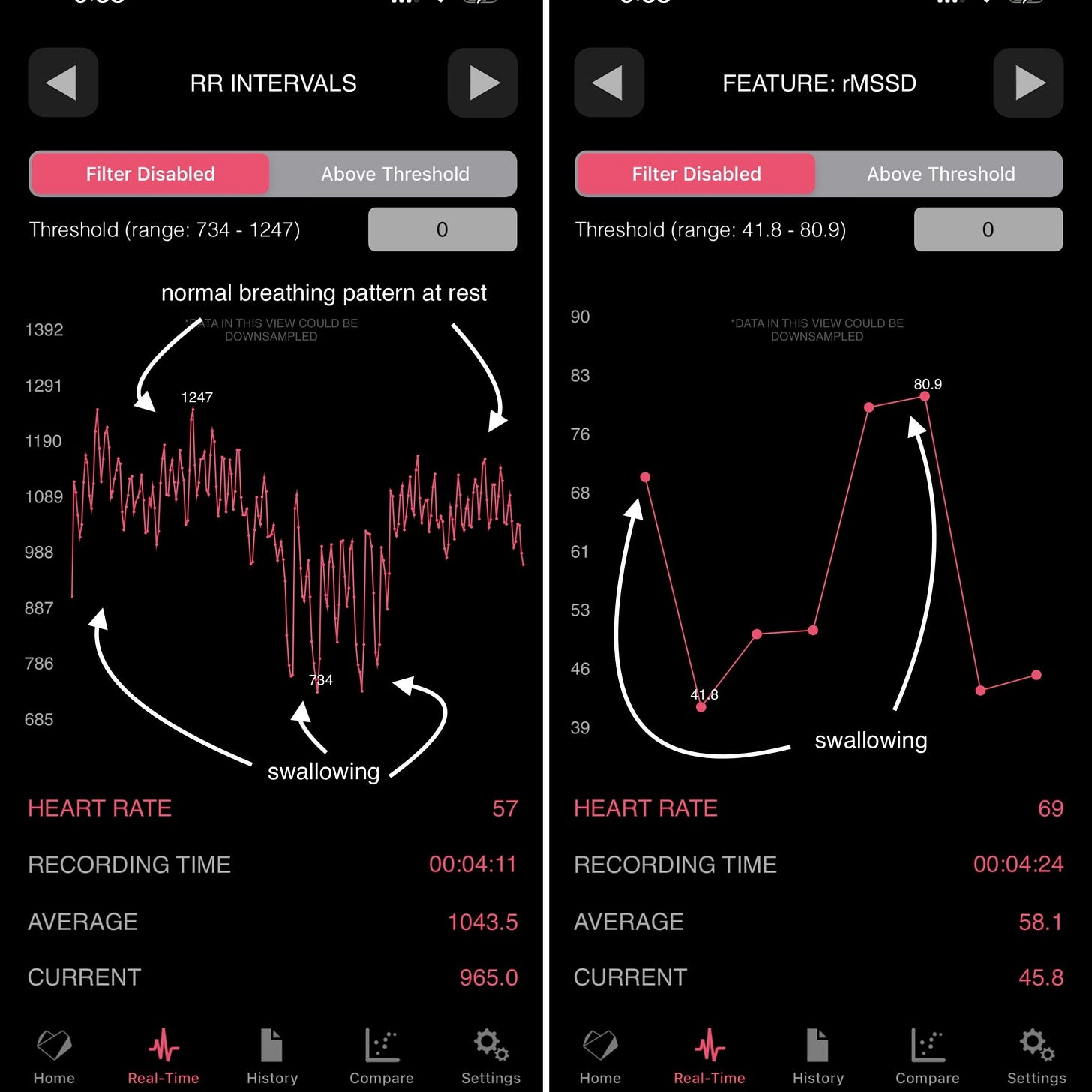
HRV when swallowing saliva is twice what it really was, at rest, when forcing myself not to swallow saliva:
actual HRV: ~45 ms
HRV when swallowing saliva: 80 ms
This has - of course - nothing to do with "stress", it is an artifact. Now consider that a healthy human will swallow spontaneously 18-400 times an hour - it is hardly possible to find a single minute of HRV data that is collected that is not just an artifact, because of swallowing.
A "real-time stress monitor" would, of course, detect lower stress or relaxation or whatever you want to call it, in the minutes in which my HRV doubles.
Below is an example from published literature, showing the same, i.e. a much higher heart rate variability (in this case quantified as RSA), with swallowing (full text of the paper here):
The relationship between HRV and stress is valid only under certain circumstances: outside of those circumstances, HRV is impacted by many other factors that have nothing to do with how stressed you are.
Use good tools and good protocols, look at the actual data (never at “stress estimates”), and you will be able to use HRV more meaningfully.
Talking
Another activity that dramatically impacts HRV is talking. This is quite obvious as talking breaks the regular breathing pattern. Below you can see some data in which HRV goes from the actual state (about 50 ms) to near or above 100 ms, just because a few sentences were spoken.
Once again, unusable data for “real-time stress monitors”.
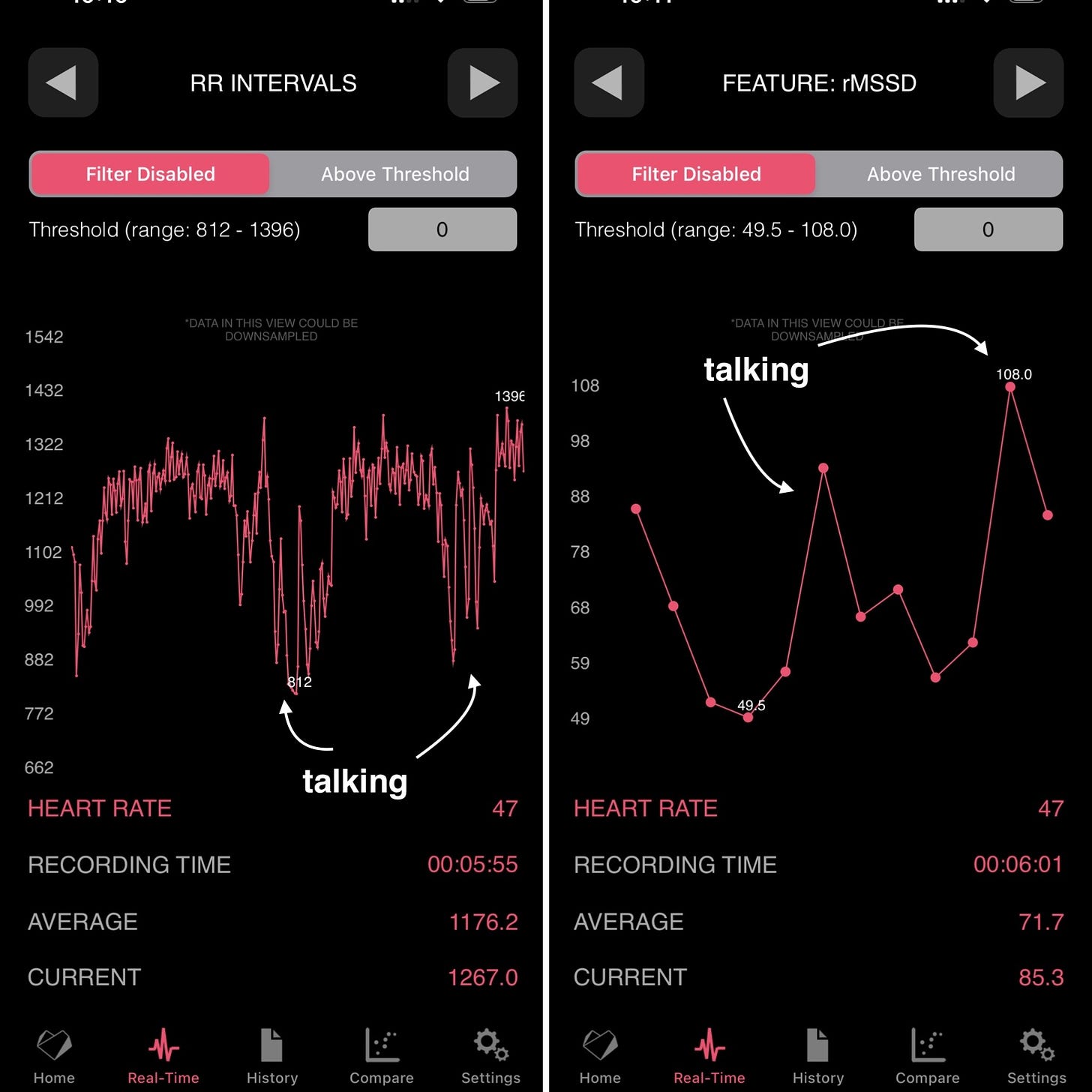
What then?
Here I have listed a few of the most obvious, but the amount of confounding factors is endless, making it so that more data (i.e. data collected outside of very well-structured protocols) does not lead to more insights, but quite the contrary.
Otherwise, just swallow saliva at a very high frequency, or talk, and you will be super-recovered ... (this sounds like nonsense, but it is exactly how people are using HRV - i.e. by looking at how artifacted or de-contextualized data results in certain HRV values, and then assuming things are “good” or “bad”, without any understanding of the physiology, the difference between acute and chronic responses, or even the ability of a device to actually capture high-quality data outside of conditions of complete rest).
Protocols were not invented to make our lives more complex. We use protocols because at times, they are the only way to meaningfully interpret collected data.
Technology
The technology for continuous HRV measurement is just not there. This is the least problematic point, as regardless of the technology, issues with physiology (discussed above) and interpretation (discussed below) will still stand.
However, it is important to stress that PPG technology, or optical measurements (the ones provided by wearables), cannot be used under conditions that are not of complete rest. Any minimal motion will trigger artifacts so large that HRV analysis cannot be carried out (even when heart rate can still be recovered with high accuracy).
Consider that just typing on your computer or slightly contracting a muscle makes HRV data collected from wearables completely unusable, artificially increasing HRV 3+ times, just because of artifacts.
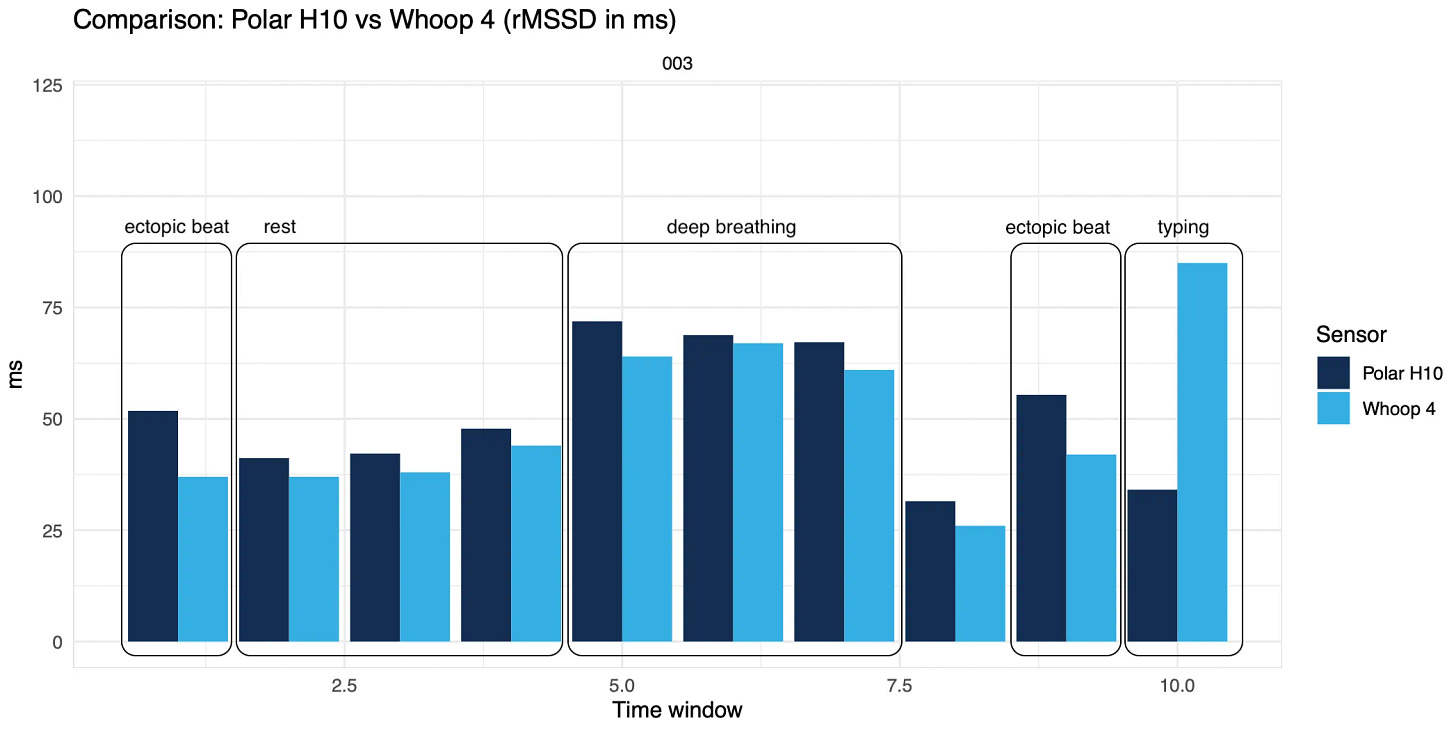
Check out the video below showing real-time PPG data while typing. Unusable, and we are talking about a very low-intensity activity, I am just sitting here writing.
Finally, the relationship between HRV (actual changes in heart activity) and PRV (changes in pulse rate variability at the finger, wrist, or other location due to volumetric changes in blood flow) is strong only under certain circumstances, again of complete rest and natural breathing. Outside of these settings, PRV, which is what you get from wearables, might depend more strongly on other factors (e.g. blood pressure, arterial stiffness, etc.).
Ask your favorite wearable to show you the raw PPG data during daily life. You’d stopped relying on it in a second if only you could see it.
HRV analysis is too prone to error for continuous measurements under conditions in which there is any motion, making the measurement unreliable (and I’m not even getting into artifacts, ectopic beats, or other cardiac abnormalities, something that up to 75% of the population experiences, which would also lead to inaccurate data at rest or during the night).
When using chest straps or other sensors able to measure the electrical activity of the heart, this is less of a problem, as they tend to be able to provide more accurate data on most occasions. However, these are typically not the systems people are willing to wear 24/7 (or that are heavily marketed to them).
If you still had doubts about how bad wearables are at continuous HRV data, and how much they are fooling you with 24/7 “stress estimates”, a recent paper (full text here) looking at raw data from one of the major manufacturers, shows the following:
Whoop’s coverage dropped from 77%–88% during the night (far from ideal) to 19%–31% during the day (no use for this data, as a single artifact in HRV data can change the data 2-3x).
Errors and variability were exacerbated by movement and posture changes during the day (almost twice the error during the day wrt the night), limits of agreement were from -32 ms to +26 ms.
Interpretation
HRV is our stress response, when measured at the right time. Measured at the right time means: 1) at rest and 2) far from stressors. Why? Measuring during or close to stressors turns any normal physiological response into something pathological (this is what ‘real time stress monitors’ in wearables are doing). In reality, it is perfectly normal for physiology to be affected for a while during and after exercise or other stressors. This is why I advocate for morning measurements, not even night data: morning measurements allow us to measure our resting physiology as far as possible from the previous day’s stressors and after the restorative effect of sleep, and as such, I consider them the ideal way to capture our stress response. A late dinner, late exercise or simply having more carbs for dinner, something we might be doing on a heavy training block, might result in increased heart rate for several hours during sleep, which is no problem but would be captured as a negative response by wearables measuring during the night. Often, night data is more tightly coupled with our behavior than with our stress response, and therefore is less useful for daily guidance.
Analyzing HRV continuously, even when done with sensors that can provide accurate data (i.e. chest straps), and in situations in which the data might reflect parasympathetic activity (e.g. at rest, with normal breathing, etc.), amplifies these issues and is often trivialized due to the disconnect between acute and chronic responses to stressors.
The simplest example here is exercise: acutely, exercise reduces HRV greatly both during and after exercise. Chronically, exercise will lead to a number of positive effects for your health, and might even increase your HRV. Similar considerations could be made for sauna or hot baths. The last time I had one, my Garmin went crazy because of the increased heart rate, a perfectly normal physiological response (blood vessels dilate to cool you off, blood pressure reduces, heart rate increases. HRV simply reduces as HR increases). People look at the data and worry. Similarly, I had several people reaching out and sharing Garmin screenshots, worried about how these devices would detect long periods of stress post-exercise, basically turning a normal physiological response into something pathological.
While this example is obvious, remember that no wearable will ever have the required context to understand what is going on beyond the most simplistic interpretations. While these issues are present also when taking resting measurements, they are amplified by continuous measurements. For stressors such as diet / food types, social interactions, etc. - the data you will see will simply highlight a somewhat aroused state and will have nothing to do with your stress response, and most importantly nothing to do with a positive or negative interpretation, your health or your performance (and this even assumes the data is not just noise, which is what you are actually collecting with your wearable).
Another example in which we had a clear decoupling between overly simplistic interpretations of HRV and our health is food intake. The best way to make a continuous stress monitor or “body battery” happy is to stop eating. Not eating triggers a sort of energy conservation mode that typically results in a much lower resting heart rate, and higher HRV. Bring this to the extreme, and you have very high HRV typically reported in people with anorexia. Is that a good health state then?
Acute and chronic responses can often differ, and continuous interpretation of HRV is often trivialized as “low HRV is bad” or “high HRV is good”, potentially leading to an unhealthy obsession with any sort of stressor, even the ones that are actually positive (or negative).
Even if we were to understand that low isn’t always bad, how do we discriminate which stressors are negative only acutely, and which ones are not, for stressors that are not as obvious as exercise? Think about how people might use HRV in the context of diet or else.
Wrap-up
The utility of HRV is in measuring our stress response, i.e. what happens in our body hours after the stressors. That’s why it is useful, if we respond well, it normalizes and is stable, if it stays suppressed, something went wrong or other stressors played a role.
Measuring in a known context (e.g. first thing in the morning, hours after stressors, and after the restorative effect of sleep), allows us to capture just that: the response. This makes the data meaningful and actionable (see an example below).
Measuring HRV all the time provides noisy, de-contextualized, and meaningless data (e.g. not linked to parasympathetic activity), risking becoming a tool to turn any normal physiological response into something pathological.
No amount of data can make up for poor protocols (or lack of protocols, as in automatically-collected data).
When it comes to HRV, less is more.
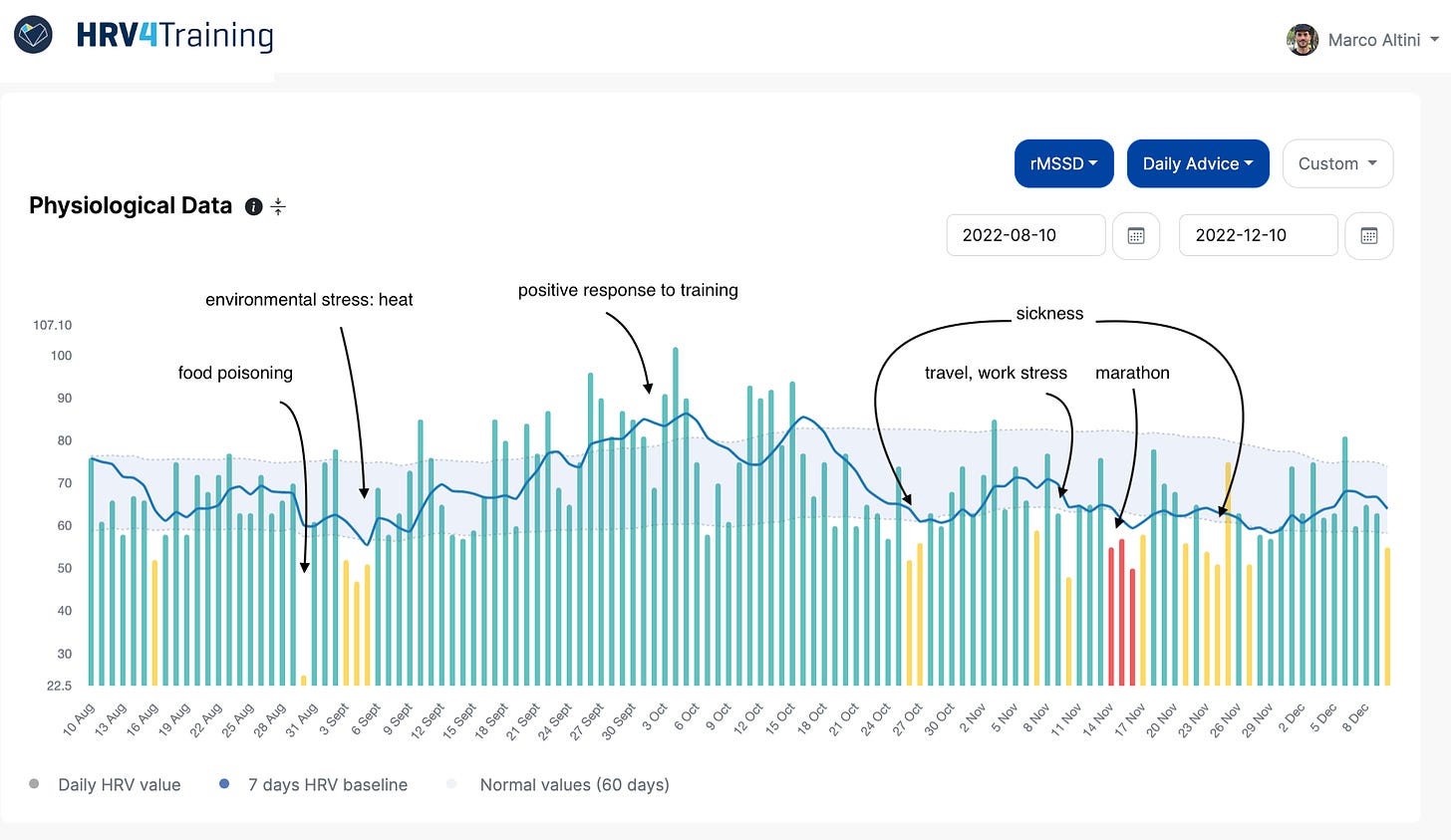
I hope this was informative, and thank you for reading!
Marco holds a PhD cum laude in applied machine learning, a M.Sc. cum laude in computer science engineering, and a M.Sc. cum laude in human movement sciences and high-performance coaching.
He has published more than 50 papers and patents at the intersection between physiology, health, technology, and human performance.
He is co-founder of HRV4Training, advisor at Oura, guest lecturer at VU Amsterdam, and editor for IEEE Pervasive Computing Magazine. He loves running.
Social:
Thank you for your insights Marco, always appreciate them! I recently got a Garmin watch and HRV was one of the many things I wanted the watch and its analytics to help me with. I had downloaded and used (not frequently I must admit) the HRV4Training app, since I wanted to get familiar with its use and applications to use with my athletes. However, as I mentioned, I wasn't too diligent with it (I wake up kind of in a hurry every day to coach an early class). Anyway, I think the HRV status from Garmin is pretty useful, albeit imperfect as you mention, since it may consider some "extra" information from the entire night, but the average of the whole night would still change from night to night based on suppression from stress. I realize sleeping HRV may be less useful than sitting or standing HRV after sleep, but I believe you can still notice the same patterns and signals (although they might be a little less clear because of the noise). Maybe I'm just trying to justify myself, what do you think?
Thank you Marco a very interesting and balanced perspective. I am fascinated by my bodies response to stressors particularly the differences created by physiological vs pathological stimulus as I try to age gracefully. It’s been an evolving journey as I have moved from a purely knowledge based perspective to a more wisdom based one creating a more appreciative interpretation. Marco you have been a valuable guide for me on that journey. Sam wearables of any brand provide so much more than HRV data, they even tell the time I wouldn’t be without mine.