Long term trends in resting heart rate and heart rate variability (HRV)
research and practical application
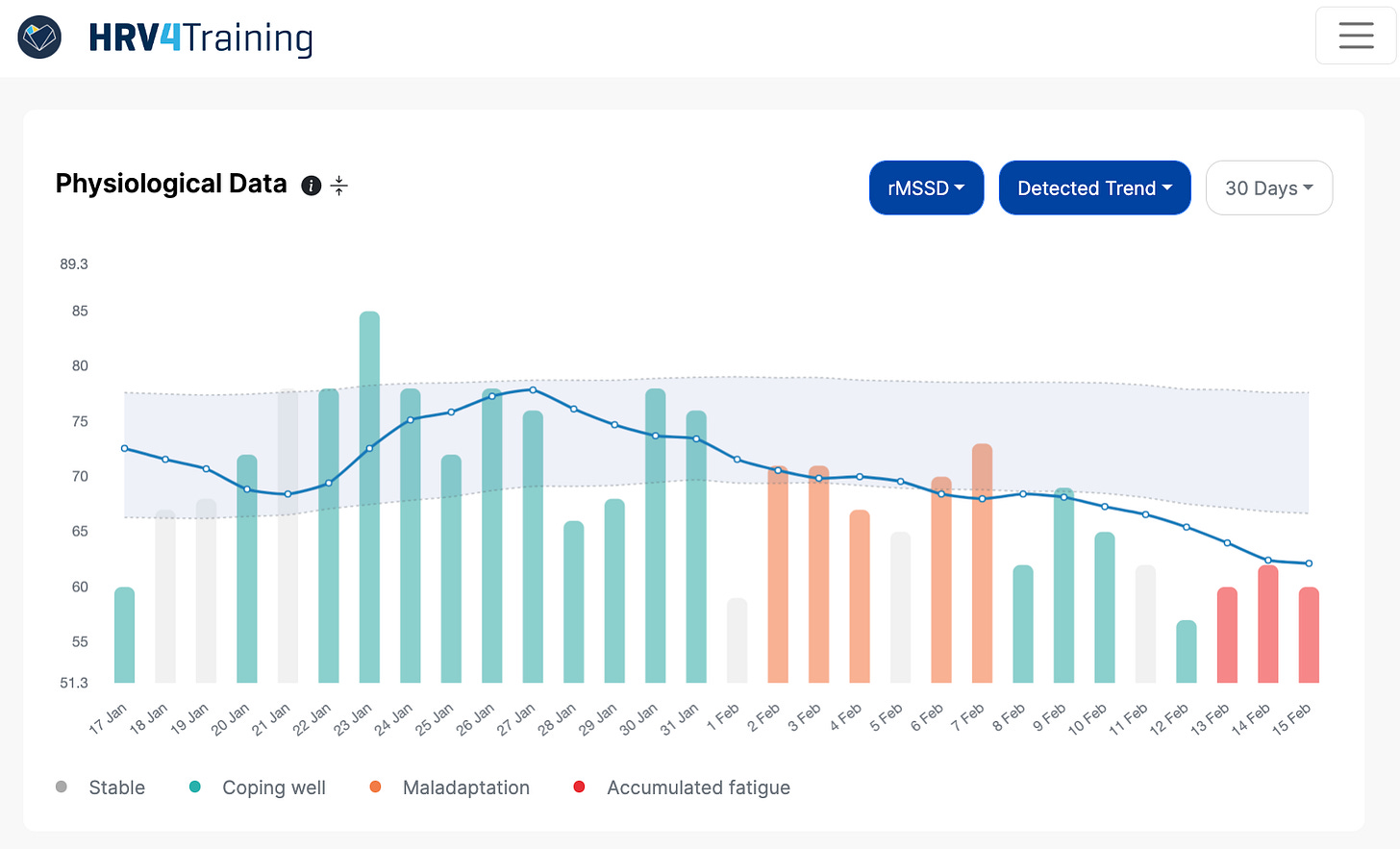
Heart rate variability (HRV) trends over long periods of time (e.g. from weeks to months) are one of the most interesting and complex aspects to analyze when it comes to resting physiology.
Analyzing our responses acutely (e.g. right after a stressor) shows clear patterns, however, there is often a difference between the short (or acute) and long-term (or chronic) effects of a stressor. For example, our HRV immediately after a hard session will reduce (acute effect, see also this blog). However, a positive response to that session, as well as other factors resulting from consistent training, even when part of a high-intensity or volume block, should result in a stable or even higher HRV in the long run (chronic effect, or trend, as discussed in the same blog).
In this post, I will cover the current research on trends and our analysis in HRV4Training, hoping it will help you make sense of the data in the longer term.
The importance of trends
Day-to-day HRV guidance can be useful to make small changes to our overall training plan. If today we are down, we can easily adapt and move our intense training by a day, or make some other small adjustments to better balance the different stressors we face. However, this kind of analysis cannot say much about the big picture. How is our overall condition? How are we responding to a new training phase? Are we at risk of non-functional overreaching or maladaptation? Analyzing trends can help you answer these questions and provide a better understanding of our overall physiological response.
Note that while most research on HRV-guided training these days relies on trends and your normal range, this is not to say that acute changes should be ignored. Acute reductions can signal incoming sickness, and should always be contextualized with your own subjective feel and all the additional information you have available. There’s a place for analyzing both acute and chronic responses if we understand the differences and are able to properly contextualize the data (some additional tips, here).
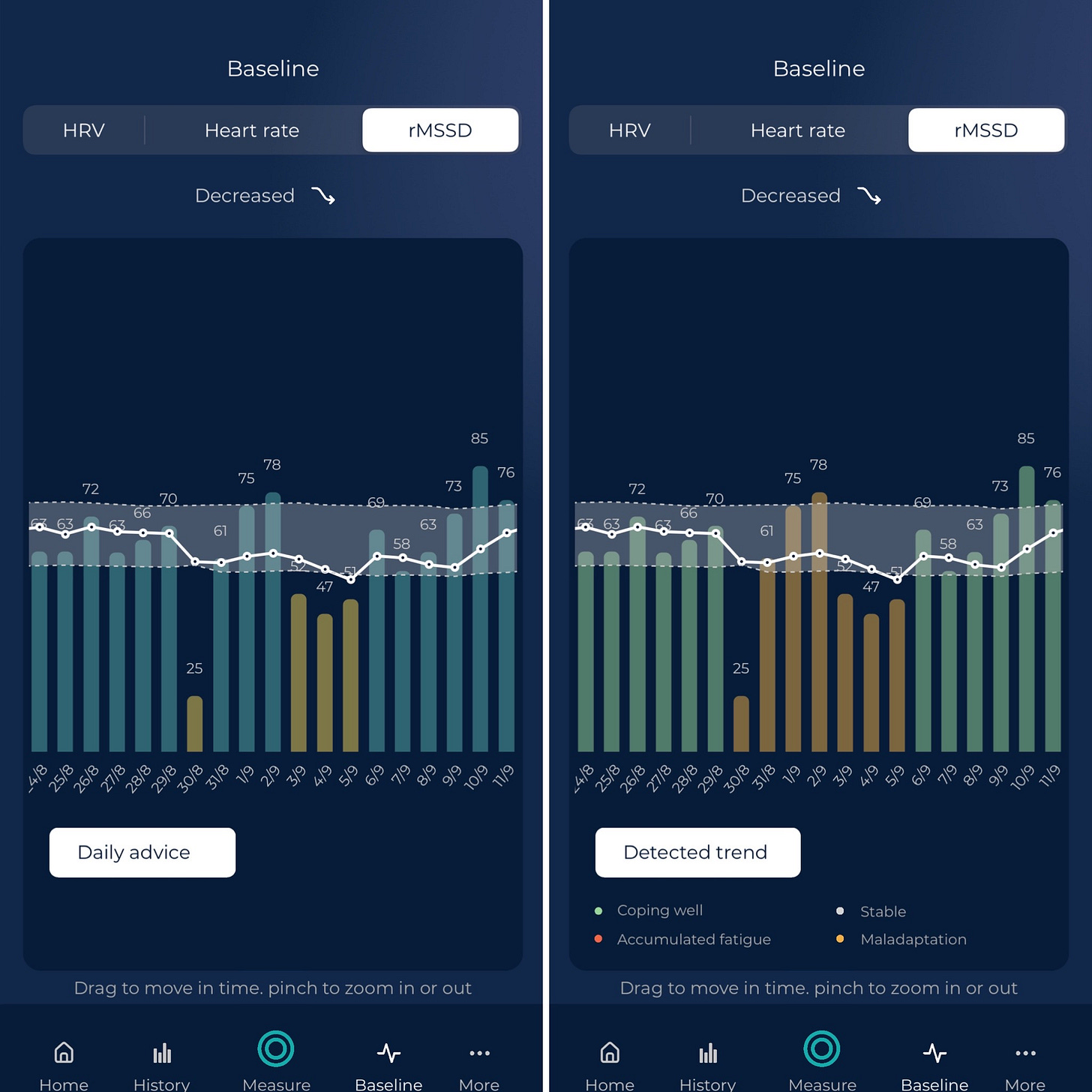
Research overview
In this section, I cover the main findings I could derive from published literature and structure them in a way that can ease trends interpretation. This post builds on a great paper published by Martin Buchheit [3], in which he already provided a generalization of the main trends seen in years of his research in sports and performance. I have combined his analysis with additional findings and considerations derived from the work of Daniel Plews [1, 6], Andrew Flatt [9], and others. On top of this, I have added my own considerations and ideas on how all of these parameters can be analyzed together, which is what I ended up implementing in HRV4Training.
It is clear from the scientific literature and my experience that looking at HRV alone will not be sufficient to understand long-term responses. Thus, in HRV4Training, we use resting heart rate, HRV, and the HRV coefficient of variation, for our trends analysis [1, 3]. Additionally, we also use the normal range, to determine if the physiological trend is causing the baseline to shift outside of what is considered normal variation for our physiology.
Let’s define the various terms and parameters we will be looking at:
Trends are changes in physiological values (e.g. heart rate, HRV) over longer periods of time, typically weeks to months. It is important to consider trends in the context of training load (e.g. high-intensity block, tapering, etc.) [5]. Our trends analysis in HRV4Training uses between 40 and 60 days of data.
When talking about HRV I am referring to rMSSD baseline values, or in other words, 7 days moving averages. The same applies to resting heart rate.
The HRV coefficient of variation is a measure of the variation in our values. Analyzing both the baseline rMSSD value and its variation can provide more insights compared to looking at the baseline only.
Normal range: your normal range is the range where we expect your data to be, provided that no abnormal stressors are present. We show the normal range on the homepage of the app, and on the Baseline page for Pro users.
Below I describe the typical patterns reported in the scientific literature, which I summarize in the next section as well.
Typically, coping well with training is associated with higher HRV and lower resting heart rate [2, 8], while not coping well is associated with the opposite. Analyzing both HRV and heart rate can be useful to better understand fatigue since a decrease in HRV alone is not necessarily associated with increased fatigue [6]. An example could be parasympathetic saturation, which results in reductions in HRV and resting heart rate (i.e. parasympathetic activity is actually increasing even if we see reduced HRV) [3]. This is a less common situation, which I discuss here.
In the average population or in recreational athletes negative adaptations to training (e.g. non-functional overreaching) are generally associated with reductions in parasympathetic activity (HRV), while better fitness and performance are typically associated with higher values of HRV [4]. In elite athletes, when training load is close to an individual maximal load, HRV can either be unchanged or reduced [6] and can be associated with both total load and specific training intensity distribution. For example, when spending more time above the second lactate threshold, HRV tends to decrease, while it tends to increase when more time is spent below the first lactate threshold or to be unchanged when spending time at moderate intensity (between thresholds) [7].
When both heart rate and HRV are stable, the coefficient of variation can be particularly useful. In particular, a reduction in the coefficient of variation has been associated with an increased risk of non-functional overreaching [1]. This makes sense since less variation might mean that there is more stress or that the system is unable to respond or bounce back. However, there is some controversy on this point since other authors interpret a reduction in HRV CV as a measure of better adaptation [9]. This also makes sense since we expect more day-to-day changes if we are not coping very well with the training and therefore HRV jumps around more, showing difficulty in maintaining homeostasis (e.g. when we start a new training phase with more high-intensity work). A multiparameter approach allows you to make sense of both trends, as a lower coefficient of variation becomes problematic only when HRV is also suppressed.
Putting it all together
We’ve seen in the scientific literature that looking at multiple parameters together is a must in the context of trying to understand long-term trends. In HRV4Training, I have implemented the following parameters, which I report here together with a basic interpretation of their use:
HRV (ln rMSSD): an increase is typically associated with coping well with training and improved fitness level. A reduction is not necessarily bad, it could be associated with parasympathetic saturation or tapering. Looking at heart rate can help figure out the different situations. Most importantly, HRV should always be considered in the context of a specific training phase. For example, an increase in HRV during a high-load phase is typical when good athletes are responding well to the stimulus and might be associated with other physiological mechanisms such as increased blood plasma volume. Such an increase in HRV during high-volume training can be considered a sign of functional overreaching, that’s an ideal response. A reduction in HRV outside of tapering and with stable or increasing resting heart rate is typically a sign of a poor physiological response to either acute or chronic stressors.
Resting heart rate: in general, an increase is associated with more fatigue if acute, or less fitness if chronic unless it is occurring during tapering. A reduction is most of the time associated with coping well with training and better cardiorespiratory fitness level, especially if we talk about trends analyzed over several weeks or months. A stable trend is ideal. Note that seasonal patterns are present (e.g. resting heart rate tends to be lower in summer), and therefore changes should always be analyzed with respect to your normal values, as we do in the HRV4Training app.
Coefficient of variation of HRV: A decrease associated with higher HRV and lower resting heart rate can be representative of coping well with training, while a decrease associated with lower HRV is probably representative of the risk of non-functional overreaching. An increase in the coefficient of variation might reflect some trouble in adapting to a new training block (or other stressors, such as travel or altitude) and if associated with reduced HRV might be a warning sign of inappropriate training load.
Normal range: one of the difficulties of analyzing trends is due to the choices we need to make in terms of the amount of data used for this analysis. For example, after a negative response, resulting in a reduction in HRV, we might have stable trends, but still far off from our normal range. This is why I included also the normal range in our multiparameter trends analysis, so that periods of long, abnormal responses, can be better quantified.
Automated trend analysis in HRV4Training
The algorithm we implemented reports one of the following conditions, based on the parameters listed above, and an analysis of how they are changing in the past 2 weeks, with respect to normal variability in our own historical data, in the previous 60 days:
Stable: values are fluctuating normally but without strong changes. Normally, this means that you can proceed as planned, but keep an eye on any acute drops.
Coping well with training: typically associated with unchanged or increased HRV and unchanged or decreased resting heart rate, together with a lower coefficient of variation, within your normal range. This means that it’s all good, and you should proceed according to your plans.
Maladaptation to training: associated with increased resting heart rate and coefficient of variation, reductions in HRV. This is a sign of a poor response to current stressors (training or other), and typically it would be a good idea to reduce training intensity or prioritize recovery in other ways.
Accumulated fatigue: decreased HRV and increased resting heart rate, with reduced coefficient of variation or HRV suppressed below your normal values. This is what is shown above for my own data, and is often associated with longer periods of poor health or poor responses that require slowing down and trying to prioritize recovery for several days or weeks.
Saturation: saturation is often associated with reduced HRV and resting heart rate, in particular for individuals with very low resting heart rates or elite athletes. It is important to analyze this trend in the context of a training program and training history. This is an experimental feature present only online in HRV4Training Pro, under the resting physiology analysis.
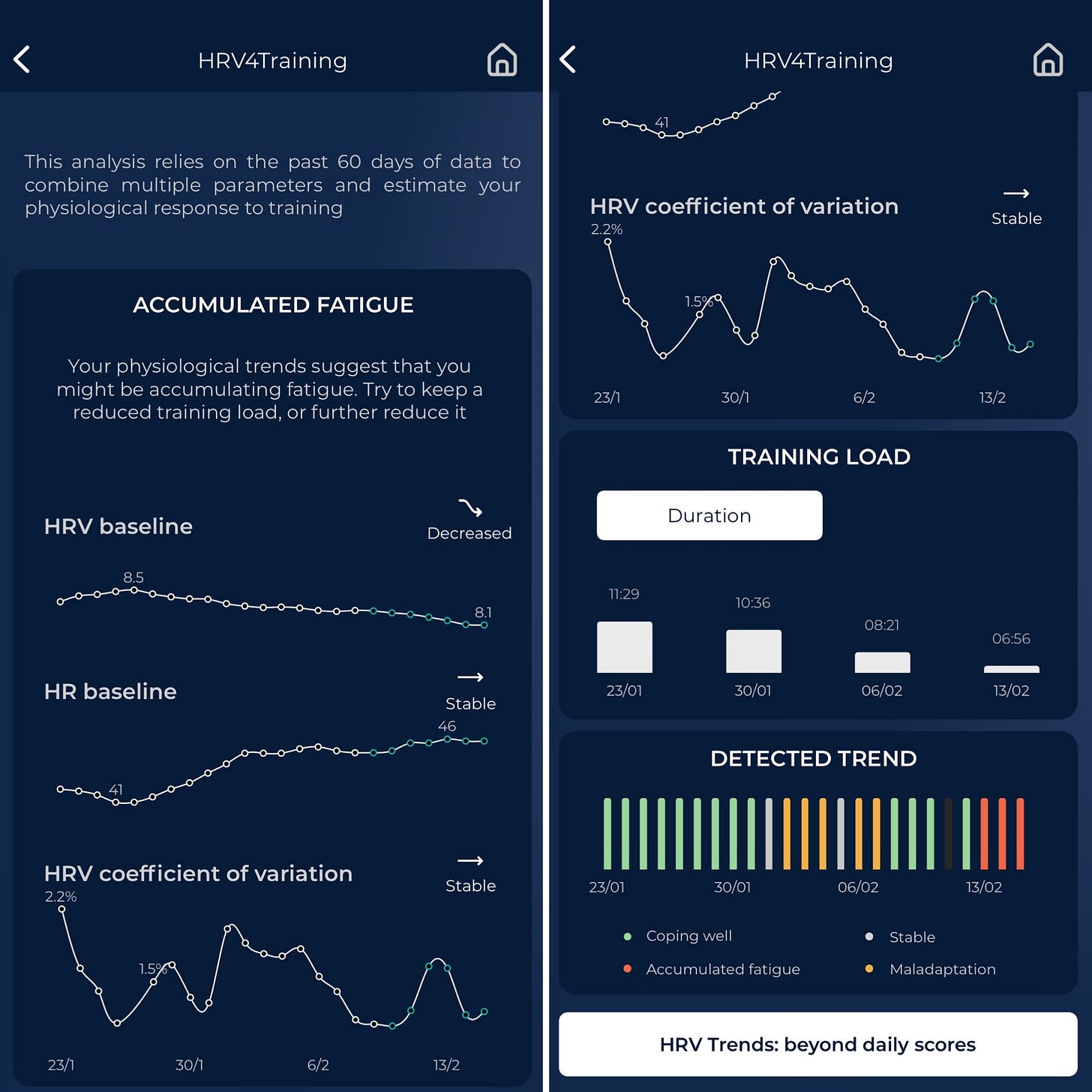
Summary
In this post, I’ve introduced the main parameters and findings reported in the scientific literature in the context of analyzing physiological data (resting heart rate, HRV, coefficient of variation) for longer-term trends.
By looking at trends, we can better understand the big picture and how different training phases and other stressors are affecting our physiology beyond acute day-to-day changes.
This analysis is part of HRV4Training, as shown in some of the screenshots above.
I hope this was informative, and thank you for reading!
Marco holds a PhD cum laude in applied machine learning, a M.Sc. cum laude in computer science engineering, and a M.Sc. cum laude in human movement sciences and high-performance coaching.
He has published more than 50 papers and patents at the intersection between physiology, health, technology, and human performance.
He is co-founder of HRV4Training, advisor at Oura, guest lecturer at VU Amsterdam, and editor for IEEE Pervasive Computing Magazine. He loves running.
Social:
[1] Plews, D. J., Laursen, P. B., Kilding, A. E., & Buchheit, M. (2012). Heart rate variability in elite triathletes, is variation in variability the key to effective training? A case comparison. European journal of applied physiology, 112(11), 3729–3741.
[2] Stanley, Jamie, Shaun D’Auria, and Martin Buchheit. “Cardiac Parasympathetic Activity and Race Performance: An Elite Triathlete Case Study.” IJSPP 10.4 (2015).
[3] Buchheit, M. (2014). Monitoring training status with HR measures: do all roads lead to Rome?. Frontiers in physiology, 5. Chicago
[4] Buchheit, M., Chivot, A., Parouty, J., Mercier, D., Al Haddad, H., Laursen, P. B., & Ahmaidi, S. (2010). Monitoring endurance running performance using cardiac parasympathetic function. European journal of applied physiology, 108(6), 1153–1167.
[5] Pichot, V., Roche, F., Gaspoz, J. M., Enjolras, F., Antoniadis, A., Minini, P. & Barthelemy, J. C. (2000). Relation between heart rate variability and training load in middle-distance runners. Medicine and science in sports and exercise,32(10), 1729–1736.
[6] Plews, D. J., Laursen, P. B., Stanley, J., Kilding, A. E., & Buchheit, M. (2013). Training adaptation and heart rate variability in elite endurance athletes: Opening the door to effective monitoring. Sports Medicine, 43(9), 773–781.
[7] Plews, D. J., Laursen, P. B., Kilding, A. E., & Buchheit, M. (2014). Heart Rate Variability and Training Intensity Distribution in Elite Rowers. International journal of sports physiology and performance.
[8] Stanley, J., D’Auria, S., & Buchheit, M. (2015). Cardiac Parasympathetic Activity and Race Performance: An Elite Triathlete Case Study. IJSPP, 10(4).
[9] Andrew Flatt blog. http://hrvtraining.com/2015/05/19/hrv-stress-and-training-adaptation/